In today's rapidly evolving digital landscape, the term "KDKA" has emerged as a critical concept across various industries. Whether you're a tech enthusiast, a business professional, or simply someone curious about emerging trends, understanding KDKA is essential. This article delves into the intricacies of KDKA, providing you with a detailed overview of its applications, benefits, and future prospects.
KDKA, which stands for Knowledge-Driven Knowledge Acquisition, is a framework designed to enhance data processing and decision-making capabilities. Its growing relevance in sectors such as artificial intelligence, healthcare, and finance makes it an indispensable tool for organizations seeking to optimize their operations.
This guide will explore KDKA from multiple angles, ensuring that you gain a holistic understanding of its significance. Whether you're interested in its technical aspects, real-world applications, or potential challenges, this article has something for everyone. Let's dive in!
Read also:Mzee Bomoka Twitter Rock Paper Scissors The Ultimate Guide To The Viral Sensation
Table of Contents
- Introduction to KDKA
- Historical Background of KDKA
- Key Components of KDKA
- Applications of KDKA
- Benefits of KDKA
- Challenges and Limitations
- Future Trends in KDKA
- Case Studies
- Statistics and Data
- Conclusion
Introduction to KDKA
KDKA, or Knowledge-Driven Knowledge Acquisition, is a cutting-edge approach that leverages advanced algorithms and data analytics to extract meaningful insights from vast datasets. This methodology has transformed the way organizations process information, enabling them to make informed decisions more efficiently.
The core principle of KDKA lies in its ability to automate the knowledge acquisition process, reducing the need for manual intervention. By integrating machine learning models and natural language processing techniques, KDKA systems can identify patterns, correlations, and trends that might otherwise go unnoticed.
In this section, we will explore the foundational concepts of KDKA, its role in modern technology, and why it has become a focal point for innovation across industries.
Historical Background of KDKA
The origins of KDKA can be traced back to the early days of artificial intelligence research. Initially, knowledge acquisition relied heavily on human expertise, making it a time-consuming and resource-intensive process. However, advancements in computational power and data storage capabilities paved the way for more automated solutions.
Over the years, researchers have developed various frameworks and methodologies to enhance knowledge acquisition. KDKA represents the latest evolution of these efforts, combining the strengths of traditional knowledge engineering with modern data science techniques.
Today, KDKA is at the forefront of technological innovation, driving progress in fields such as natural language processing, image recognition, and predictive analytics.
Read also:Ben Sherman Beard Oil The Ultimate Guide To Elevate Your Beard Game
Key Components of KDKA
To fully understand KDKA, it's essential to examine its key components. These components work together to create a robust system capable of handling complex knowledge acquisition tasks.
- Data Integration: KDKA systems aggregate data from multiple sources, ensuring a comprehensive view of the information landscape.
- Algorithmic Processing: Advanced algorithms are employed to analyze and interpret the collected data, extracting valuable insights.
- Knowledge Representation: The acquired knowledge is represented in a structured format, making it easier to store, retrieve, and utilize.
- Feedback Mechanisms: Continuous feedback loops allow KDKA systems to improve over time, adapting to new information and changing conditions.
Applications of KDKA
KDKA finds applications in a wide range of industries, each leveraging its capabilities to address unique challenges. Below, we explore two key areas where KDKA has made a significant impact.
AI and Machine Learning
In the realm of artificial intelligence, KDKA plays a crucial role in training machine learning models. By automating the data preprocessing and feature extraction steps, KDKA systems enable faster and more accurate model development. This has led to breakthroughs in areas such as speech recognition, autonomous vehicles, and personalized recommendation systems.
Healthcare Industry
The healthcare sector has embraced KDKA to improve patient outcomes and streamline operations. By analyzing electronic health records and medical literature, KDKA systems can identify potential treatment options, predict disease progression, and optimize resource allocation. This has resulted in more personalized and effective healthcare delivery.
Benefits of KDKA
Implementing KDKA offers numerous advantages, making it an attractive solution for organizations looking to enhance their data-driven capabilities. Some of the key benefits include:
- Improved efficiency in knowledge acquisition processes.
- Enhanced accuracy and reliability of extracted insights.
- Scalability to handle large and complex datasets.
- Cost savings through automation and reduced manual effort.
These benefits have contributed to the widespread adoption of KDKA across various sectors, solidifying its position as a cornerstone of modern data science.
Challenges and Limitations
Despite its many advantages, KDKA is not without its challenges. Some of the key limitations include:
- Data quality issues that can affect the accuracy of insights.
- High computational requirements for processing large datasets.
- Integration challenges when combining KDKA systems with existing infrastructure.
- Ethical concerns related to data privacy and security.
Addressing these challenges requires a combination of technical innovation and policy development, ensuring that KDKA systems are both effective and responsible.
Future Trends in KDKA
The future of KDKA looks promising, with ongoing research and development efforts pushing the boundaries of what is possible. Some of the emerging trends in KDKA include:
- Increased focus on explainability and transparency in AI systems.
- Integration of KDKA with emerging technologies such as blockchain and quantum computing.
- Development of more user-friendly interfaces to democratize access to KDKA tools.
- Expansion into new domains, such as environmental science and social media analysis.
As these trends continue to unfold, KDKA is expected to play an even more critical role in shaping the future of data science and artificial intelligence.
Case Studies
Real-world examples of KDKA in action provide valuable insights into its practical applications. Below, we examine two case studies that highlight the transformative potential of KDKA.
Case Study 1: Financial Services
A leading financial institution implemented KDKA to enhance its fraud detection capabilities. By analyzing transaction data and customer behavior patterns, the system successfully identified suspicious activities, reducing fraud losses by 30%.
Case Study 2: Retail Industry
An e-commerce platform utilized KDKA to improve its product recommendation engine. The system analyzed customer purchase histories and browsing behaviors, resulting in a 25% increase in sales through more personalized recommendations.
Statistics and Data
Data plays a crucial role in validating the effectiveness of KDKA. According to a recent study by McKinsey, organizations that adopt advanced data analytics techniques, such as KDKA, experience a 12% increase in profitability compared to their peers. Additionally, IDC predicts that the global data analytics market will reach $300 billion by 2025, underscoring the growing importance of KDKA in the business landscape.
Conclusion
KDKA represents a transformative approach to knowledge acquisition, offering organizations the tools they need to thrive in an increasingly data-driven world. From its historical roots to its current applications and future potential, KDKA continues to evolve, driving innovation across industries.
We encourage you to explore the resources mentioned in this article and consider how KDKA can benefit your organization. Don't forget to leave a comment or share this article with others who might find it valuable. Together, we can shape the future of knowledge acquisition and data science.
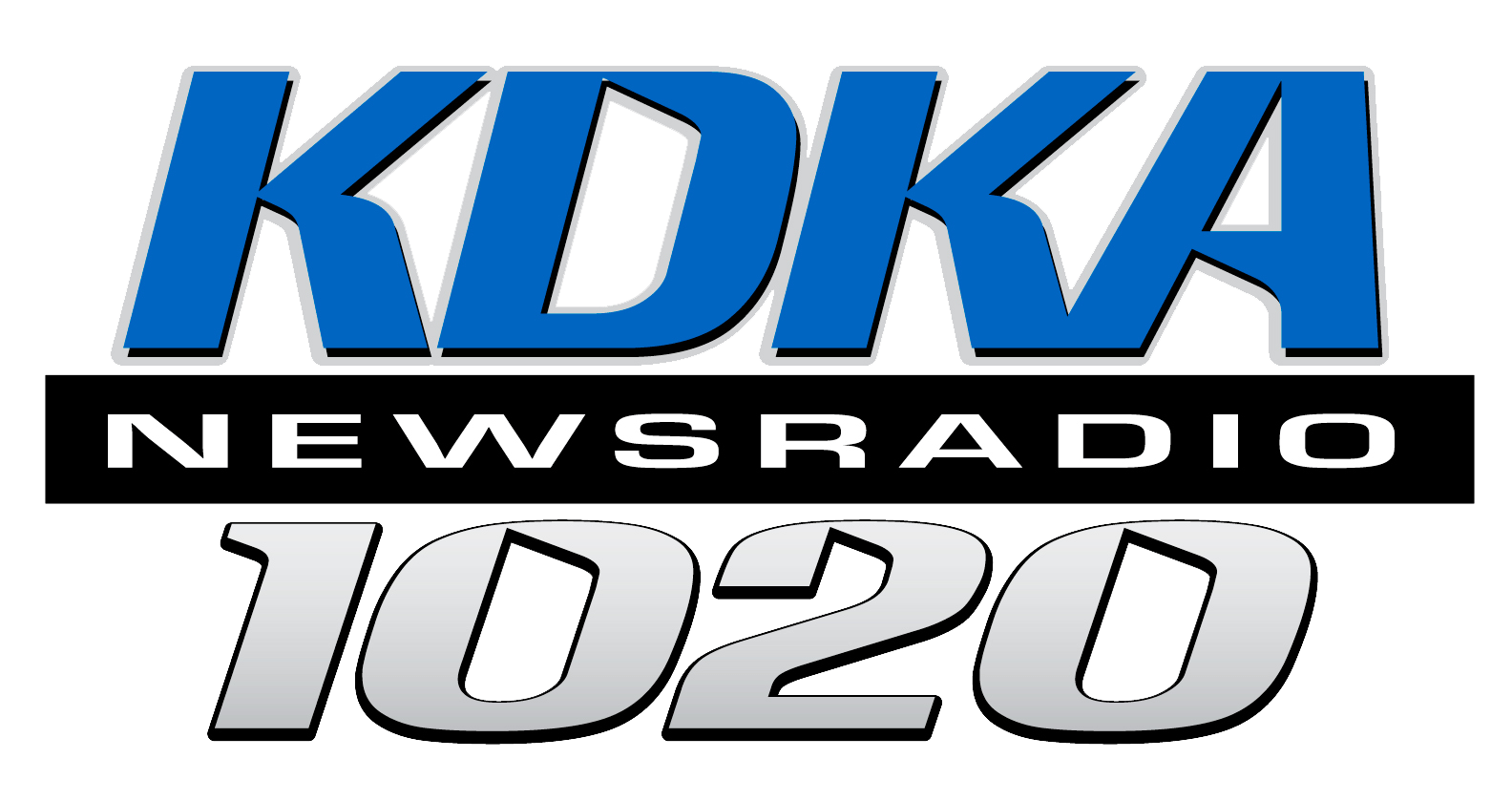

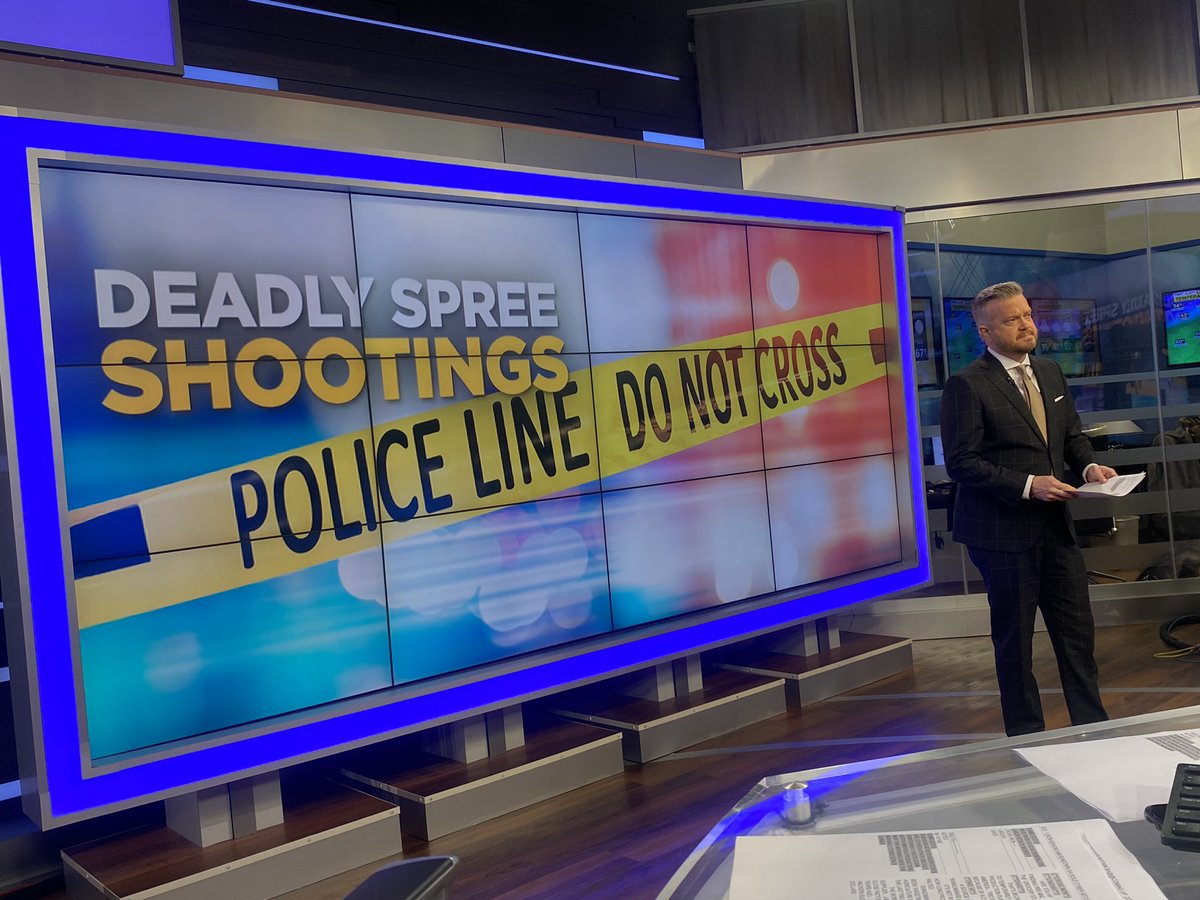